Understanding Top-Down and Bottom-Up Ethics in AI Part 2
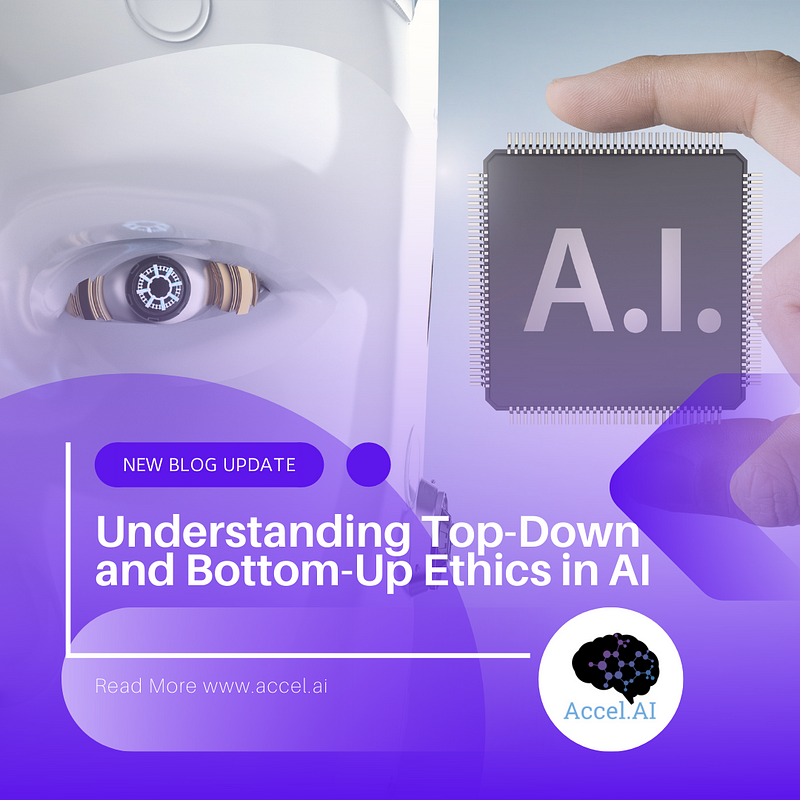
In part two of this investigation into top-down and bottom-up ethics in Artificial Intelligence (AI), I would like to explore three different angles, including the technical perspective, the ethical viewpoint, and through a political lens while also discussing individual and hybrid approaches to implementation.
The first angle is to understand the technicalperspective, broken down into programming and applied machine learning: Essentially how to implement algorithmic policies with balanced data that will lead to fair and desirable outcomes.
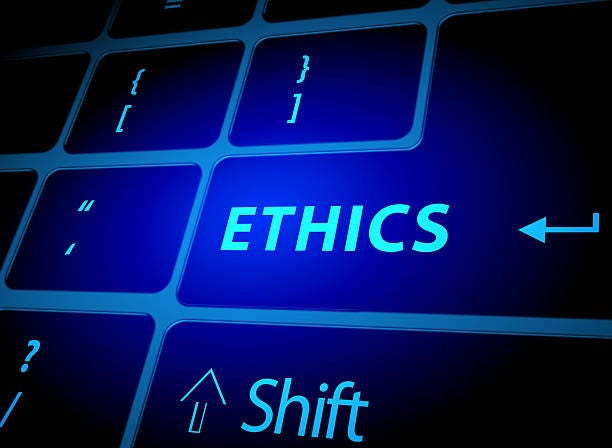
The next angle is the theoretical ethics viewpoint: Ethics can work from the top-down, coming from rules, philosophies, etc., or bottom-up looking at the behaviors of people and what is socially acceptable for individuals as well as groups, which varies by culture.
Third, I want to come back to my original hypothesis that top-down implied ethics dictated from the powers that be, and bottom-up could only be derived from the demands of the people. We might call this a more political perspective.
Finally, we will connect them all back together and split them apart again, into top-down, bottom-up, and hybrid models of how ethics functions for AI. This is an exercise in exploration to reach a deeper understanding. How ethics for AI works, in reality, is a blend of all of these theories and ideas acting on and in conjunction with one another.
Technical Machine Learning Top-Down vs Bottom-Up
The technical angle of this debate is admittedly the most foreign to me, however, in my research, I have found some basic examples that I hope are helpful.
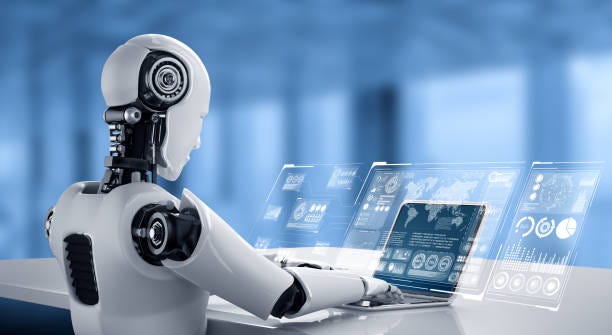
“In simple terms and in the context of AI, it is probably easiest to imagine ‘Top-down AI’ to be based on a decision tree. For example, a call center chatbot is based on a defined set of options and, depending on the user input, it guides the caller through a tree of options. What we typically refer to as AI these days — for applications such as self-driving cars or diagnostic systems in health care — would be defined as ‘Bottom-up AI’ and is based on machine learning (ML) or deep learning (DL). These are applications of AI that provide systems with the ability to automatically learn and improve from experience without being explicitly programmed.” (Eckart, 2020)
Top-down systems of learning can be very useful for some tasks that machines can be programmed to do, like the call center example above. However, if they are not monitored, they could make mistakes and it is up to us people to catch those mistakes and correct them. They may also lack exposure to sufficient data to make a decision or prediction in order to solve a problem, leading to system failure. This is the value of having a ‘human in the loop’. This gets more complicated when we move into the more theoretical world of ethics.
Bottom-up basically defines machine learning. The system is given data to learn from, and it uses that information from the past to predict and make decisions for the future. This can work quite well for many tasks. It can also have a lot of flaws built-in because the world that it learns from is flawed. We can look at the classic example of harmful bias being learned and applied, for instance in who gets a job or a loan, because the data from the past reflects biased systems in our society.
Here we will mention the use of a hybrid model of top-down and bottom-up, that has a base of rules or instructions, but then also is fed data to learn from as it goes. This method claims to be the best of both worlds and covers some of the shortcomings of both top-down and bottom-up models. For instance, self-driving cars can be programmed with laws and rules of the road, and also can learn from observing human drivers.
Theoretical Ethics Top-Down vs Bottom-Up
Now let’s move on to talk about Ethics. The first thing we need to mention in this part of the analysis is that ethics has been historically made for people, and people are complex in how they understand and apply ethics, especially top-down ethics.
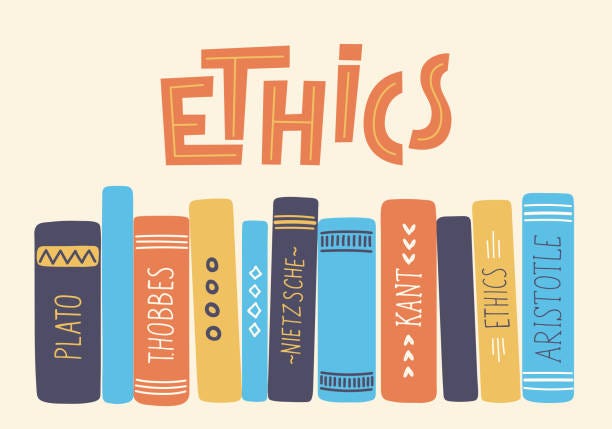
“Top-down ethical systems come from a variety of sources including religion, philosophy, and literature. Examples include the Golden Rule, the Ten Commandments, consequentialist or utilitarian ethics, Kant’s moral imperative and other duty-based theories, legal codes, Aristotle’s virtues, and Asimov’s three laws for robots.” (Wallach et. al, 2005)
The one exception on this list that doesn’t apply to people is of course Asimov’s laws, which are applied precisely for AI. However, Asimov himself said that they were flawed.
“When thinking of rules for robots, Asimov’s laws come immediately to mind. On the surface, these three laws, plus a ‘zeroth’ law that he added in 1985 to place humanity’s interest above that of any individual, appear to be intuitive, straightforward, and general enough in scope to capture a broad array of ethical concerns. But in story after story Asimov demonstrates problems of prioritization and potential deadlock inherent in implementing even this small set of rules (Clark, 1994). Apparently, Asimov concluded that his laws would not work, and other theorists have extended this conclusion to encompass any rule-based ethical system implemented in AI (Lang, 2002).” (Wallach et. al, 2005)
… Asimov concluded that his laws would not work, and other theorists have extended this conclusion to encompass any rule-based ethical system implemented in AI.
A lot of science fiction doesn’t predict the future as much as warn us against its possibilities. Furthermore, the top-down approach is tricky for AI in different ways than how it is tricky for humans.
As humans, we learn ethics as we go, from those practiced by our families and community, how we react to our environment, and how others react to us. One paper made the case that “. . . while one can argue that individuals make moral choices on the basis of this or that philosophy, actual humans first acquire moral values from those who raise them, and then modify these values as they are exposed to various inputs from new groups, cultures, and subcultures, gradually developing their own personal moral mix.” (Etzioni, 2017)
This personal moral mix could be thought of as a hybrid model for ethics for humans. The question is, how easy and practical is it to take human ethics and apply them to machines?
Political Ethics Top-Down vs Bottom-Up
When I hear top-down, I imagine either government or big Business/big Tech figureheads, sitting in a room making decisions for everyone else. This has always put a bad taste in my mouth. It is how our world works, in some ways more than others, but we are also seeing it with how big Tech has approached ethics in AI.
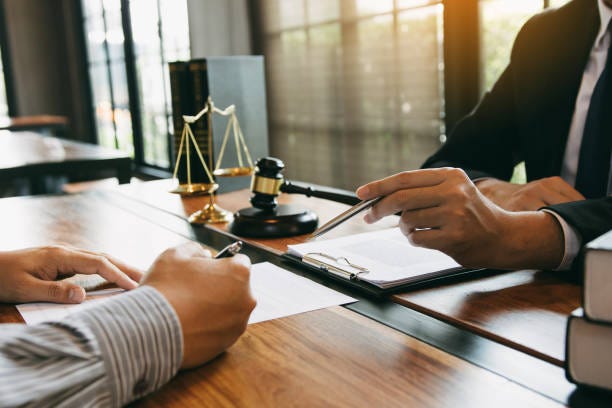
Here are some examples of top-down ethics from the powers that be: “The Asilomar AI principles, developed in 2017 in conjunction with the Asilomar conference for Beneficial AI, outline guidelines on how research should be conducted, ethics and values that use of AI must respect, and important considerations for thinking about long-term issues (Future of Life Institute 2017). . . Around the same time, the US Association for Computing Machinery (ACM) issued a statement and set of seven principles for Algorithmic Transparency and Accountability, addressing a narrower but closely related set of issues (ACM US Public Policy Council 2017).” (Whittlestone et al. 2019)
We are also seeing some crowd-collected considerations about ethics in AI, and this is what I think of when I hear bottom-up: decisions being called for by the people. This is the grassroots ethics that I think we need to be paying attention to, especially the voices of marginalized and minoritized groups.
“Bottom-up data institutions are seen by some as mechanisms that could be revolutionary for rebalancing power between big tech corporations and communities. It was argued that there is a widespread assumption that bottom-up data institutions will always be benign and will represent everyone in society, and these assumptions underpin their promotion. It was discussed whether bottom-up data institutions are, by definition, only representative of the particular communities included within their data subjects rather than of general societal values.” (ODI, 2021)
This is an important point to keep in mind when thinking about bottom-up and grassroots ethics: there will always be different ethics coming from different groups of people, and the details of the applications of it are where the disagreements abound.
The Top-Down Method of AI Being Taught Ethics
Now we get to recombine all of the top-down angles together: The technical, the theoretical, and the political.
If we teach AI ethical core principles and expect it to live by human values and virtues, I imagine we will be sorely disappointed. There just isn’t a foreseeable way to make this work for everyone.
“Many of the principles proposed in AI ethics are too broad to be action-guiding. For example, ensuring that AI is used for “social good” or “the benefit of humanity” is a common thread among all sets of principles. These are phrases on which a great majority can agree exactly because they carry with them few if any real commitments.” (Whittlestone et al. 2019)
Furthermore, if these principles are being administered from big Tech or the government, there could be a lot that slips by because it sounds good. In my previous article, we were working with the example of fairness. Fairness is something we can all agree is good, but we can’t all agree on what it means in practice. Fair for one person or group could equate to really unfair to another.
“The strength of top-down theories lies in their defining ethical goals with a breadth that subsumes countless specific challenges. But this strength can come at a price: either the goals are defined so vaguely or abstractly that their meaning and application are subject for debate, or they get defined in a manner that is static and fails to accommodate or may even be hostile to new conditions.” (Wallach et. al, 2005)
A machine doesn’t implicitly know what ‘fairness’ means. So how can we teach it a singular definition when fairness holds a different context for everyone?
A machine doesn’t implicitly know what ‘fairness’ means. So how can we teach it a singular definition when fairness holds a different context for everyone?
The Bottom-up Method of AI Being Taught Ethics
The bottom-up approach isn’t as easy to wrap up. Sometimes we see crowd-sourced (bottom-up politically) principles (top-down theoretically) being called for, and possibly a hybrid model (technically) for applied AI. If it was purely bottom-up and learning from the ethics of people, I fear disappointment will be the end result. We as humans haven’t quite mastered ethics, let alone standardized it into something codifiable.
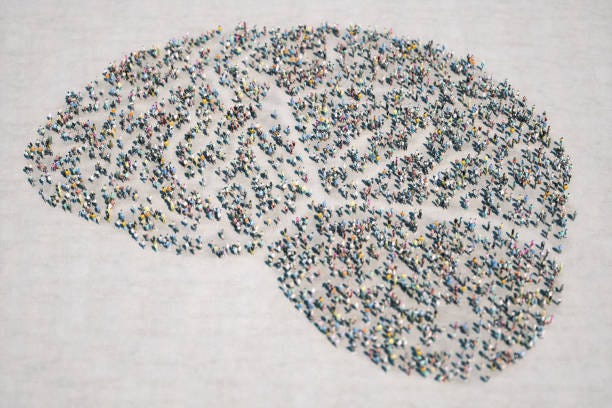
One paper describes bottom-up approaches as “. . . those that do not impose a specific moral theory, but which seek to provide environments in which appropriate behavior is selected or rewarded. These approaches to the development of moral sensibility entail piecemeal learning through experience, either by unconscious mechanistic trial and failure of evolution, the tinkering of programmers or engineers as they encounter new challenges or the educational development of a learning machine.” (Allen et. al. 2005)
This is very very challenging and time-consuming. And as we know, AI doesn’t learn as humans do. It lacks a solid foundation. Building on top of that and applying band-aid after band-aid is not going to help.
“Bottom-up strategies hold the promise of giving rise to skills and standards that are integral to the overall design of the system, but they are extremely difficult to evolve or develop. Evolution and learning are filled with trial and error — learning from mistakes and unsuccessful strategies. This can be a slow task, even in the accelerated world of computer processing and evolutionary algorithms.” (Allen et. al. 2005)
The Hybrid of Bottom-Up and Top-Down Ethics for AI
So if top-down is flawed and bottom-up isn’t promising, what about a hybrid model? “If no single approach meets the criteria for designating an artificial entity as a moral agent, then some hybrid will be necessary. Hybrid approaches pose the additional problems of meshing both diverse philosophies and dissimilar architectures.” (Allen et. al. 2005)
According to most experts, a hybrid model is the better choice. Rules and structure are helpful, but only to a point, and sometimes, they contradict each other. AI is good at following rules, but it struggles around ethics, which are subjective and often contradictory.
Bringing it All Together
We have taken apart top-down and bottom-up ethics in AI in three ways: technically, theoretically, and politically. Then we took a step back and looked at top-down, bottom-up, and hybrid models of ethics for AI. Well, it still seems pretty messy, like we need to all be doing a lot more research and work in this area, but I hope that this has been helpful to understand the various angles of this analysis. To leave us with a final thought: “Ethical issues are never solved, they are navigated and negotiated as part of the work of ethics owners.” (Moss and Metcalf, 2019)
“Ethical issues are never solved, they are navigated and negotiated as part of the work of ethics owners.”
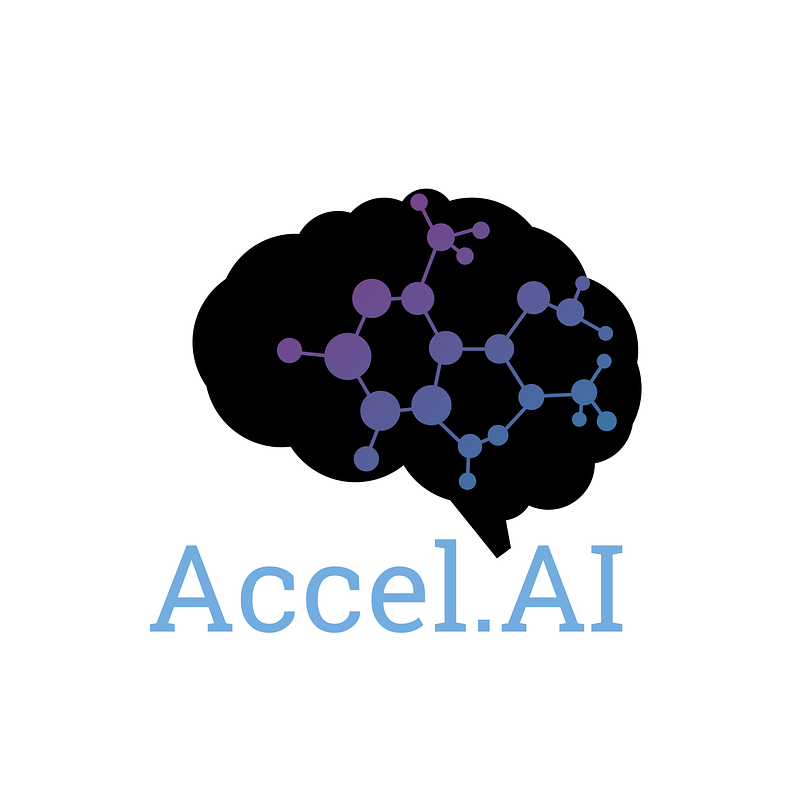
You can stay up to date with Accel.AI; workshops, research, and social impact initiatives through our website, mailing list, meetup group, Twitter, and Facebook.
Join us in driving #AI for #SocialImpact initiatives around the world!
If you enjoyed reading this, you could contribute good vibes (and help more people discover this post and our community) by hitting the 👏 below — it means a lot!
Citations
Allen, C., Wallach, W., & Smit, I. (2005). Artificial morality top-down bottom-up and hybrid approaches. Artificial Morality: Top-down, Bottom-up, and Hybrid Approaches. Retrieved December 3, 2021, from https://www.researchgate.net/profile/Wendell-Wallach/publication/225850648_Artificial_Morality_Top-down_Bottom-up_and_Hybrid_Approaches/links/02bfe50d1c8d2c733e000000/Artificial-Morality-Top-down-Bottom-up-and-Hybrid-Approaches.pdf.
Eckart, P. (2020, May 29). Top-down AI: The simpler, data-efficient AI. 10EQS. Retrieved December 13, 2021, from https://www.10eqs.com/knowledge-center/top-down-ai-or-the-simpler-data-efficient-ai/.
Etzioni, A., & Etzioni, O. (2017). Incorporating ethics into Artificial Intelligence — Philpapers. Retrieved November 30, 2021, from https://philpapers.org/archive/ETZIEI.pdf.
Google. (2021). #OPEN roundtable summary note: Experimentalism — le guin part 2. Google Docs. Retrieved December 13, 2021, from https://docs.google.com/document/d/1cMhm4Kz4y-l__2TQANClVVMLCd9X3X8qH3RfhAGghHw/edit?pli=1#.
Moss , E., & Metcalf, J. (2019, November 14). The ethical dilemma at the heart of Big Tech Companies. Harvard Business Review. Retrieved December 13, 2021, from https://hbr.org/2019/11/the-ethical-dilemma-at-the-heart-of-big-tech-companies.
Wallach, W., Smit, I., & Allen, C. (2005). Machine morality: Bottom-up and top-down approaches … — AAAI. Machine Morality: Bottom-up and Top-down Approaches for Modeling Human Moral Faculties. Retrieved December 3, 2021, from https://www.aaai.org/Papers/Symposia/Fall/2005/FS-05-06/FS05-06-015.pdf.
Whittlestone, J., Cave, S., Alexandrova, A., & Nyrup, R. (2019). The role and limits of principles in AI Ethics: Towards a … Retrieved December 13, 2021, from http://lcfi.ac.uk/media/uploads/files/AIES-19_paper_188_Whittlestone_Nyrup_Alexandrova_Cave.pdf.